In a forecasting context with uncertainties, chances are more than one reasonable forecast can be produced. Meteorology is a typical example where multiple weather models provide different forecasts. Ensemble forecasting consists in designing a better forecast that combines these different forecasts.
As an example, below are multiple yearly solar radiation maps, as given by the yearly average of daily forecasts by different weather models.
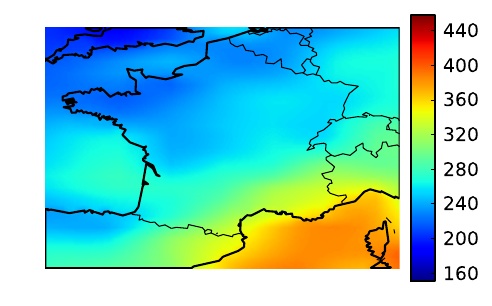
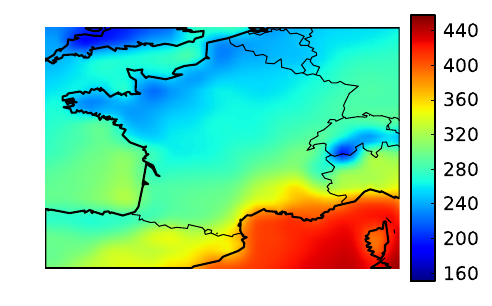
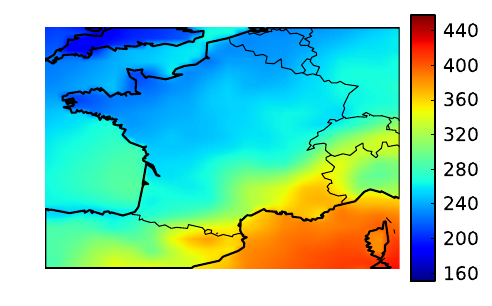
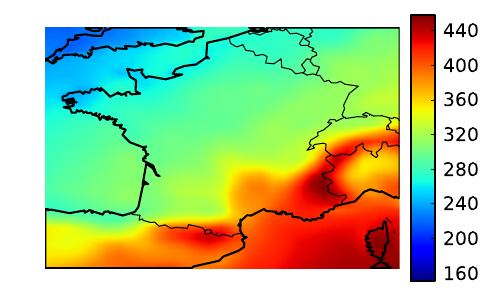
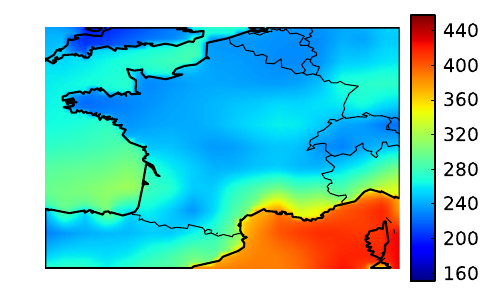
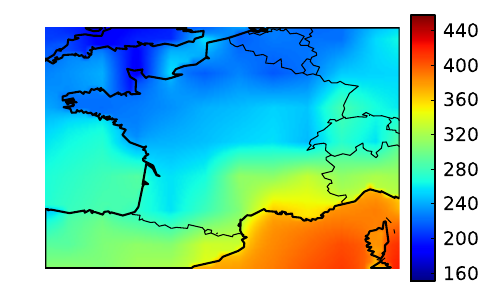
Online learning (sequential aggregation) methods can be employed to produce a new, improved forecast that combines the available forecasts and shows better performance than any of these individual forecasts. The new forecast shows very low bias as illustrated below. Also note that the ensemble forecast has higher resolution than the individual model forecasts, which is possible because independent learning is carried out at every point of the map.
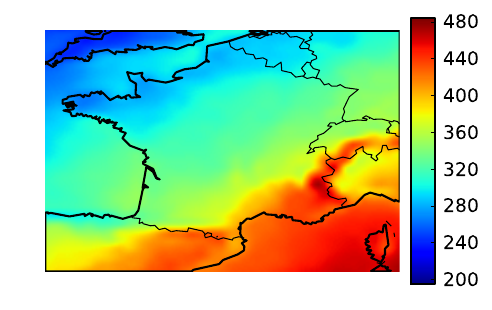
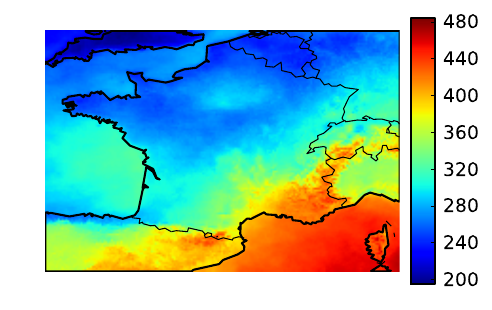
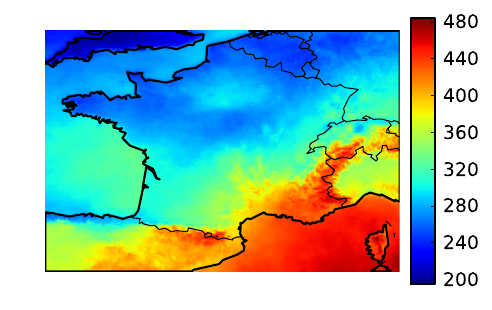
A linear combination of the individual forecasts is made with weights that are learned online and can change quickly to adapt to a new situation. The figure below is an example of the time evolution of the weights associated to each individual forecasts (in this case, 48 individual forecasts).
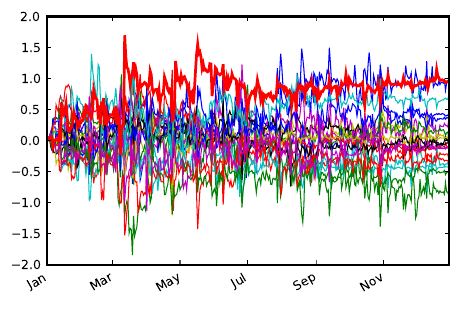
Online learning can be oriented toward probabilistic forecast so as to produce a probability density function instead of a single deterministic forecast.
Such work was applied for renewable energies at EDF (main French electricity supplier) and also integrated into the weather forecasting process of Meteo France (French national weather agency). Its implementation for renewable energies is patented under French patent FR3044138 in which I am co-inventor with my former PhD student J. Thorey.